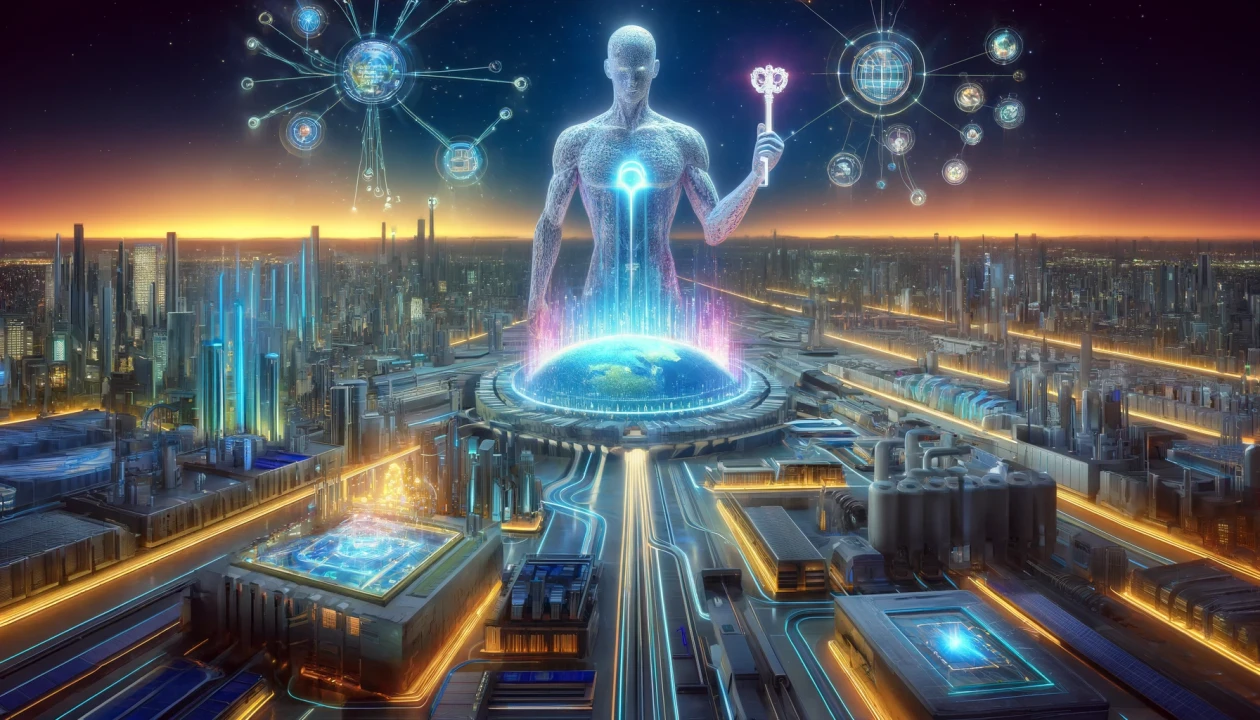
The global supply chain is a data behemoth – a sprawling network of manufacturers, suppliers, carriers, distributors, and retailers. It's a complex web of transactions often bogged down by inefficient processes and a lack of transparency. This is in part due to the way data has traditionally been managed, often trapped in silos, a clear example of this occurs with Trade Management Solutions (TMS). While these TMS platforms hold vital information, the absence of open APIs has frequently hindered both innovation and the free flow of information upon which the entire supply chain depends.
Artificial intelligence (AI), however, is poised to disrupt this status quo. Advancements in technologies like Large Language Models (LLMs), intelligent agents, and retrieval-augmented generation hold the key to unlocking these silos and bringing a new era of optimization, efficiency, and transparency to the supply chain.
Traditional TMS platforms, while undoubtedly useful, have often operated as walled gardens. Critical supply chain data resides inside these systems, accessible primarily through the TMS interface or proprietary integrations. This lack of open access has led to several challenges:
Vendor Lock-in: Companies that invest heavily in a TMS solution often become reliant upon it. Switching providers can be complicated and costly due to the time and resources required to extract data and migrate to a competing system. This can discourage innovation, as companies may avoid adopting potentially game-changing technologies if they require abandoning their current TMS.
Limited Visibility: Data silos prevent businesses from having a holistic, real-time understanding of their supply chains. Information may be scattered across multiple systems, making it difficult to track shipments, identify bottlenecks, or make data-driven decisions.
Redundancies and Increased Costs: The inability to seamlessly integrate data from a TMS with other software applications (like warehouse management or analytics tools) can lead to data duplication and manual processes. This is both inefficient and prone to error.
The emergence of powerful AI technologies is rapidly changing the landscape, making it possible to address these challenges and usher in a new age of supply chain intelligence. While the lack of digitalization in the industry was due to industry inertia and proprietary platforms, AI will unlock the silo’s of data so entities that want to move quickly will not be hindered by legacy friction. This will allow for more differentiation and faster digitalization. Here's how specific advancements will make this a reality:
1. Large Language Models (LLMs)
LLMs are a type of AI model that excels in understanding and processing natural language. They have been trained on massive amounts of text data, enabling them to communicate, generate different creative text formats, and translate languages. In the world of supply chain management, LLMs have the potential to revolutionize how data is accessed and utilized:
Intelligent Data Extraction: LLMs can analyze complex trade documents like contracts, bills of lading, and invoices, extracting key information and converting it into structured data that can be easily integrated with other systems. This eliminates the need for manual data entry, streamlines processes, and reduces errors.
Semantic Understanding and Search: LLMs power semantic search engines that understand the nuances of supply chain terminology and the relationships between concepts. This means users can pose inquiries in natural language rather than relying on rigid queries, making it much easier to find the information they need from multiple systems.
Data Interoperability: LLMs can facilitate data interoperability between different TMS platforms and other supply chain systems, acting as a translation layer. This eliminates the need for complex integrations, allowing companies to freely adopt best-of-breed solutions rather than being bound to a single monolithic system.
2. Intelligent Agents
Intelligent agents are software programs that can perform tasks autonomously on behalf of a user or system. In the supply chain, they can be harnessed to break down silos and streamline operations. Examples include:
Negotiation Agents: AI-powered agents can analyze market trends, carrier performance data, and historical rates to negotiate optimal freight contracts on behalf of businesses. They can even engage in real-time negotiations with carriers, ensuring the best possible terms.
Compliance Agents: These agents monitor complex international trade regulations, stay up-to-date on changes, and flag potential compliance issues. This minimizes risks and ensures adherence to relevant laws and regulations.
Proactive Monitoring Agents: Intelligent agents can monitor the supply chain 24/7, scanning for potential disruptions, anomalies, or trends. These might include weather disruptions, port delays, or changes in consumer demand, alerting relevant stakeholders and suggesting proactive mitigation strategies.
3. Retrieval-Augmented Generation
Retrieval-augmented generation (RAG) is a technique that combines the power of LLMs with the ability to retrieve relevant information from a vast knowledge base. In the context of the supply chain, it can enable TMS platforms and other systems to answer complex questions, provide insights, or perform tasks that would normally require a human expert:
Contextualized Decision Support: RAG models can analyze historical data, market conditions, and real-time updates to provide context-aware recommendations on freight routing, carrier selection, or inventory management. This augments decision-making capabilities far beyond what traditional TMS reporting dashboards offer.
Personalized Insights: RAG models can sift through data and tailor supply chain insights to specific users. For example, a logistics manager might receive a personalized report highlighting critical KPIs and potential bottlenecks, while a procurement officer might have a dynamic view of demand trends and optimal sourcing strategies.
Predictive Analytics: By combining retrieval and generation, RAG models can deliver more sophisticated predictive capabilities. They can identify patterns in historical data, access external data sources like news or regulatory updates, and generate detailed forecasts to aid with proactive and strategic planning.
The potential impact of AI on the supply chain is profound. These technologies will foster a shift from siloed, reactive supply chain management to proactive, collaborative, intelligence-driven optimization. The result will be:
Democratization of Data: AI will help break down silos, allowing companies of all sizes to access and leverage supply chain data more easily. This will level the playing field, opening up opportunities for smaller players and fostering a more competitive market.
Rise of Data-Driven Decision-Making: With AI providing insights and recommendations at every step, supply chain management will become less about intuition and more about informed, data-driven decision-making. This shift will lead to improved efficiency, reduced risks, and cost savings.
Innovation through Collaboration: Increased data interoperability and open APIs will encourage businesses to collaborate more effectively with suppliers, carriers, and logistics partners. This will enable the development of innovative supply chain solutions that weren't possible in the past, leading to increased resilience and agility across the board.
For companies ready to embrace the transformative power of AI, here are some steps to consider:
Invest in Data Quality: As AI models are only as good as the data they're trained on, it's critical to ensure your supply chain data is accurate, consistent, and well-structured.
Experiment with AI Pilots: Identify small-scale, focused applications where AI can be tested and evaluated on a manageable scale. It helps to build internal buy-in and pave the way for broader AI adoption.
Embrace an Open API Strategy: Look for TMS and other supply chain solutions like Openebl.org that prioritize open APIs and encourage integration. It facilitates seamless data exchange, enabling flexibility and avoiding the risk of future vendor lock-in.
Foster a Culture of Continuous Learning: AI is a rapidly evolving field. Encourage employees to stay up-to-date on the latest advancements, engage in ongoing training, and be open to new ways of working.
The integration of AI into TMS and other supply chain systems is no longer a theoretical prospect– it is an ongoing reality that is reshaping the industry. Businesses that embrace AI and adopt an open, collaborative approach will be best positioned to reap the benefits of greater efficiency, visibility, and innovation. The era of siloed supply chains is coming to an end, giving way to an intelligent, interconnected, and dynamic global trade network.
This post is originally posted on Sean O'Malley's LinkedIn.